It's not a matter of life and death, but..
- sandip amlani
- Jan 17
- 6 min read
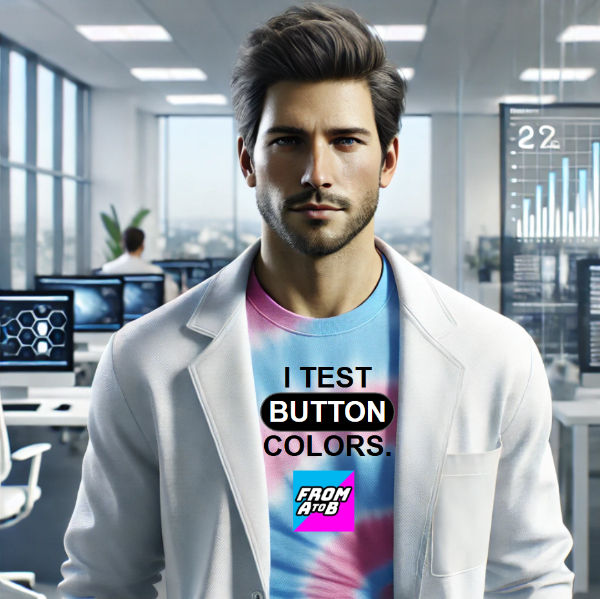
"That’s bad science"—three words every scientist hates to hear. The whole idea of what they do is to get to the truth through systematic testing and following the data. Manipulating data to fit a predefined idea? That’s not just bad practice; it’s a betrayal of the very principles science is built on.
So why should it be any different in the business world? Conversion Rate Optimisation (CRO) professionals often call themselves the “scientists of the business world,” and rightly so. We design experiments, test hypotheses, and deliver insights that drive major decisions.
But here’s the uncomfortable truth: many CROs don’t behave like scientists. From cherry-picking metrics to justify decisions to ignoring critical analysis after tests, the same bad practices that would make a scientist cringe are often shrugged off in the business world. I mean, after all, it's not a matter of life and death, right?
This post dives into the parallels between bad science and bad CRO practices, using real-world examples from both fields to illustrate what happens when governance goes out the window.
1. HARKing
(Hypothesising After Results are Known)
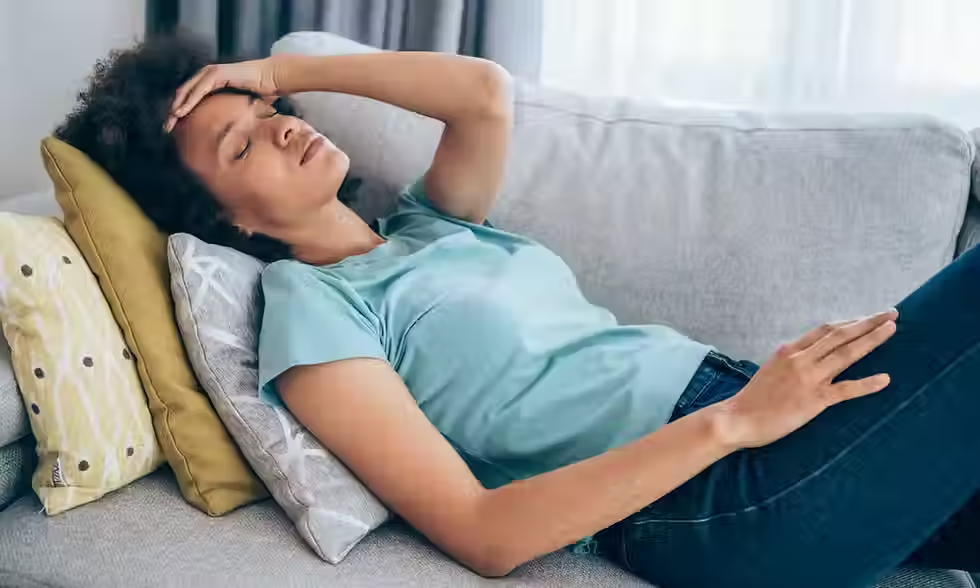
The 2011 PACE Trial on chronic fatigue syndrome (CFS) involved severe HARKing. Researchers initially hypothesised that Cognitive Behavioural Therapy (CBT) and graded exercise therapy (GET) would help patients. However, when the results didn’t support their hypothesis, the researchers altered the metrics mid-study to redefine “improvement” and “recovery.” This made the therapies appear more effective than they were. Many patients followed the advice based on the study, engaging in treatments that worsened their symptoms.
A classic example of HARKing in business is Facebook’s early experiments with ad placement. Facebook ran A/B tests to determine which ad formats and placements would drive higher engagement. However, instead of starting with clear hypotheses, the team analysed the data post-test and retroactively crafted hypotheses to explain why certain formats performed better. For instance, they attributed the success of larger ads to "improved user visibility," while smaller ads were explained as "less disruptive to user experience"—explanations that conveniently fit the observed outcomes rather than guiding the initial test design.
2. Cherry Picking Metrics
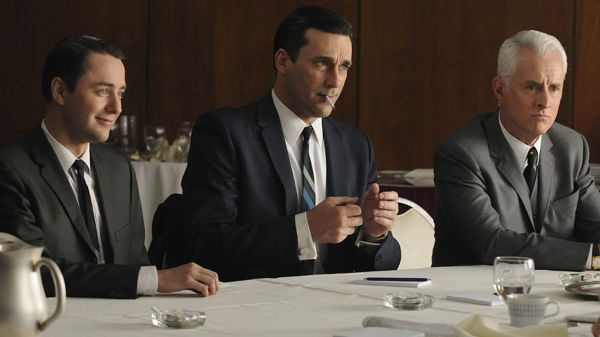
For years, the tobacco industry incentivised researchers to cherry-pick data to minimise the links between smoking and cancer. Scientists hired by the industry ignored evidence showing harm, instead publishing studies that downplayed risks. For example, a series of papers claimed that smoking was associated with health benefits such as weight loss, overshadowing clear evidence of its role in cancer and respiratory diseases.
Uber provides an example from business where focusing on vanity metrics—like clicks or sign-ups—while ignoring key business drivers lead to issues down the line. Uber’s referral bonus experiments focused on user acquisition (sign-ups) but failed to evaluate the downstream impact on profitability and masking financial losses.
3. Running Tests with Insufficient Sample Sizes
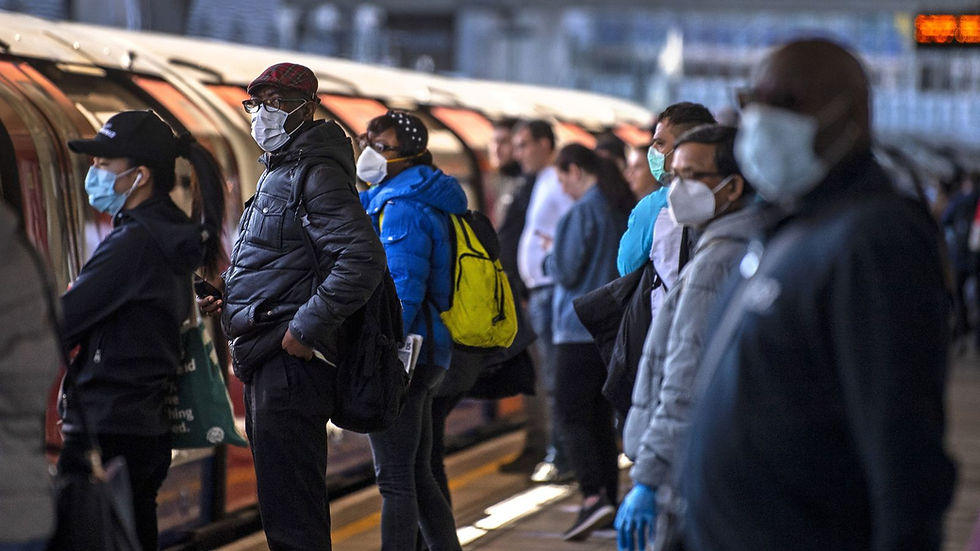
The early stages of the COVID-19 pandemic saw trials for hydroxychloroquine conducted with small sample sizes. These studies initially suggested the drug was effective against the virus, prompting widespread use, policy changes and even an endorsement by the then President Trump. However, larger and more rigorous trials later debunked these findings, revealing no significant benefits and leading to unnecessary deaths, wasted resources and public confusion. Thankfully, no scientists supported the claim that disinfectants are effective against the virus.
Groupon provides our business example of the dangers of using small sample sizes. They faced retention issues after rolling out changes based on winning tests offering deep discounts across various product categories with small sample sizes. They assumed these quick wins would scale but later realised they lacked sufficient sample sizes to assess the long-term impact on customer retention or merchant profitability leading to a downturn on both metrics.
4. P-Hacking (a.k.a Data Dredging)
(Manipulating data to find significance)
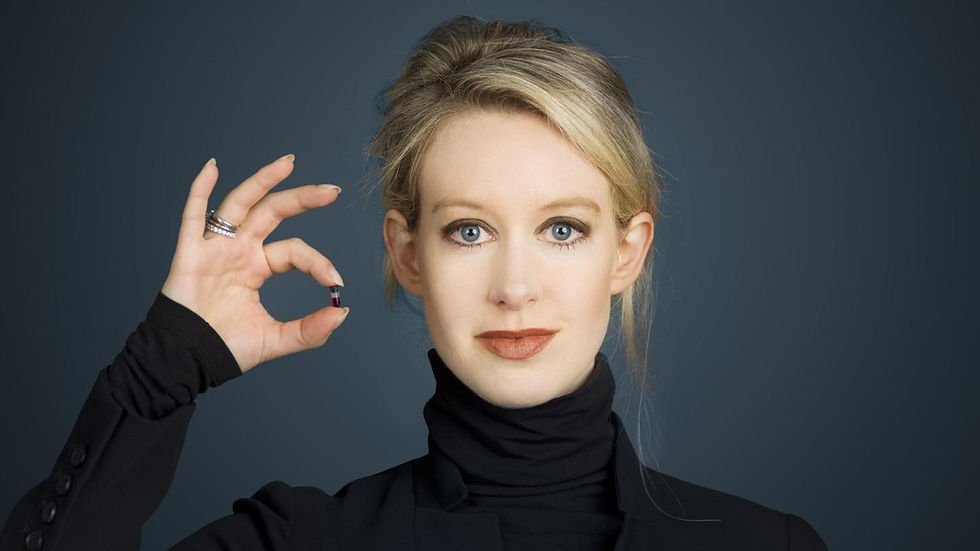
Theranos was a high growth blood-testing company that claimed to be able to perform hundreds of tests with a single drop of blood. The founder ended up in jail after lying to investors and customers about the accuracy rate and manipulating metrics from diagnostics tests. Inaccurate results included a false HIV positive, a false cancer diagnosis and a false indication of a miscarriage.
In the A/B testing world, let’s take a look at a meta analysis to highlight the impact of P-hacking. A study analysed over 2000 A/B tests on the Optimizely A/B testing platform. The findings revealed that approximately 73% of experimenters halted their tests upon achieving a 90% confidence level, a practice indicative of p-hacking. Stopping tests too early increased the false discovery rate from 33% to 40%, leading companies to implement changes based on misleading data. Such false positives resulted in an estimated 1.95% loss in expected lift, translating to significant revenue losses.
5. Ignoring Post-Test Analysis

OceanGate’s Titan submersible disaster in 2023 highlights the dangers of ignoring critical test results. The Titan used a carbon-fiber hull, a novel but controversial material for deep-sea exploration. Engineers and industry experts repeatedly raised concerns about its ability to withstand extreme pressures, urging more rigorous testing. These warnings were not headed, and in June 2023, during a dive to the Titanic wreck, the Titan submersible catastrophically imploded, resulting in the tragic loss of all five individuals on board.
PepsiCo’s launch of Crystal Pepsi in the 1990s demonstrated what can happen when teams stop at identifying a winning variation without analysing why it worked / didn't work. Early test markets showed interest in the new drink which lead to a roll out of it. However, much of this demand was purely curiosity rather than a genuinely affinity to the new product. PepsiCo didn’t analyse whether this curiosity for Crystal Pepsi would translate into sustainable long-term demand.
Spoiler alert: It didn't.
6. Not Sharing Failures
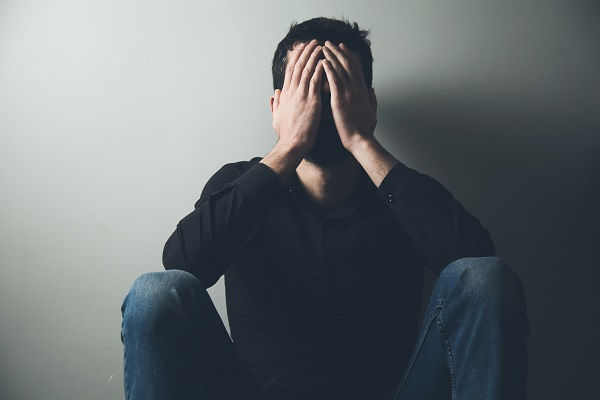
Lack of transparency around failed drug trials has repeatedly slowed medical progress. For example, trials for antidepressants often failed to show efficacy, but pharmaceutical companies withheld these results to avoid poor sales. As a result, doctors continued prescribing ineffective medications, delaying better treatments.
Volkswagen’s Dieselgate scandal illustrates the dangers of concealing failures. During the development of its “clean diesel” engines, engineers discovered that the engines couldn’t meet emissions standards while maintaining performance and fuel efficiency. Instead of sharing this issue with stakeholders and addressing the root cause, VW developed software to cheat emissions tests. This decision to hide the failure led to widespread regulatory violations, legal actions, and a massive reputation hit for the company.
7. Over-reliance on Tools
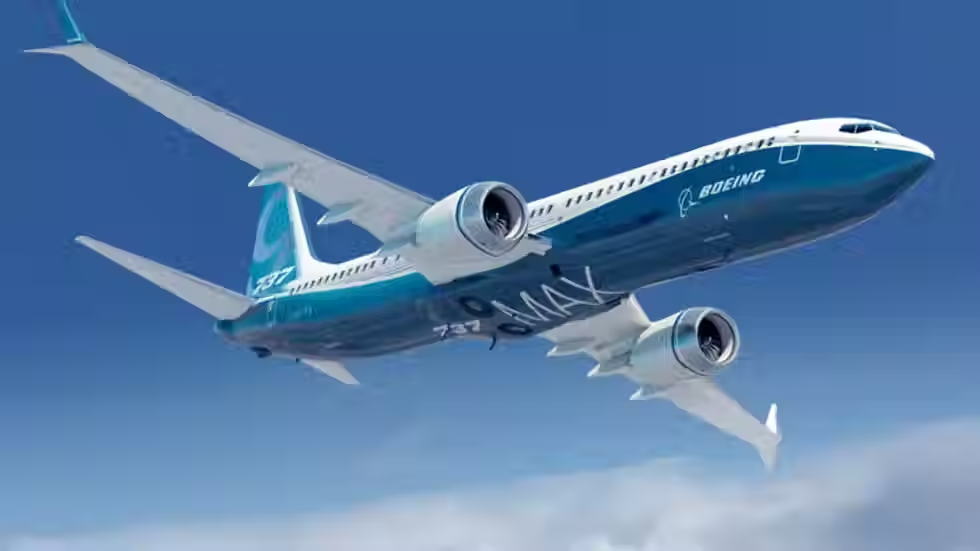
Ebay ran an experiment to evaluate the effectiveness of its paid search advertising campaigns. Over a short period, early results appeared statistically significant, suggesting that paid ads were driving a substantial number of clicks and conversions. Confident in the tool’s calculations, eBay continued to pour millions into search advertising.
However, the experiment was live for only a few days, failing to account for seasonality, natural fluctuations in user behaviour, or broader attribution challenges. When the test was later revisited with a more rigorous methodology, eBay discovered that many of the clicks attributed to paid ads were from users who would have visited the site organically. The company realised it had been overspending on search ads for years, leading to hundreds of millions of wasted dollars.
Boeing provides a business example of the catastrophic consequences of over-reliance on software and insufficient testing. Boeing wanted to get a new aircraft quickly to market to compete with Airbus. The MCAS system was created to mimic the handling of the plane’s predecessor, heavily relying on software and automation to minimise pilot retraining. However, this led to the nose of the plane automatically pointing downwards in certain circumstances, resulting in two tragic crashes, costing 346 lives.
It’s Not a Matter of Life and Death, But...
Whilst there are examples of scientists engaging in clearly dodgy practices, CRO’s must hold themselves much closer to the governance standards as (most of) our lab-coat-wearing counterparts.
Bad testing practices in business don’t usually result in the same catastrophic consequences as those in science but that doesn’t mean we should accept mediocrity. Poor governance in your CRO program can lead to wasted resources, eroded trust, and missed opportunities for growth. Doing CRO isn’t just about running tests — it’s about building a culture of experimentation that drives meaningful, long-term results for your business. For that to happen, you simply cannot skip proper governance in your CRO program.
Let’s strive for better. Let’s start behaving like the scientists of the digital world we claim to be.
What other bad practices in CRO have you come across? Drop them in the comments! 👇
Commentaires